Exploring Predictive Maintenance through Electrical Signature Analysis
Induction motors are the leading drive systems used in Industrial setups. Induction motors are used in wide load applications from low voltage to high voltage. Unplanned downtime or late fault detection in these motors can lead to following losses for the factory:
- Loss in production
- High energy consumption
- High capex investments due to reduced asset life
- Higher maintenance cost due to its unplanned nature
Vibration, Ultrasound, Thermography, Electrical Signature Analysis (ESA) and Motor Current Signature Analysis (MCSA) are some common condition monitoring techniques used for predictive maintenance of induction motors, transmission systems and loads connected to them. These are used for early diagnosis and to predict problems such as broken rotor bar, air gap eccentricity, shorted turns in low voltage stator windings, and mechanical issues. This blog sets out to present the fundamentals of Electrical Signature analysis and its benefits.
What are the benefits of an ESA based reliability or predictive maintenance system?
ESA can be used to perform regular monitoring and analysis to track and trend equipment performance and reliability. It can enable early detection of incipient induction motor, transmission and load faults. It can do this while the motor is still operational and without any requirement to stop operations. ESA powered condition monitoring and predictive maintenance of induction motors can help you achieve the following benefits:
- Early Fault Detection: ESA can identify subtle changes in electrical signatures that may indicate early signs of equipment degradation or impending failure, allowing for proactive maintenance intervention before a breakdown occurs.
- Comprehensive Monitoring: ESA provides a comprehensive approach to equipment monitoring by capturing a wide range of electrical parameters, including voltage, current, frequency, and harmonics, offering insights into the health and condition of the equipment.
- Predictive Analytics Integration: ESA data can be integrated with predictive analytics and machine learning algorithms to develop predictive maintenance models that forecast equipment failure probabilities based on historical data and trends.
- Reduced Downtime: By detecting potential faults early and scheduling maintenance proactively, ESA helps minimize unplanned downtime and production disruptions, improving overall equipment availability and productivity.
- Cost Savings: Implementing ESA as part of a predictive maintenance strategy can lead to cost savings by reducing the need for emergency repairs, extending equipment lifespan, and optimizing maintenance schedules based on actual asset condition.
- Continuous Improvement: Historical data analysis drives continuous improvement in maintenance strategies, refining predictive models for optimized performance.
How does Electrical Signature Analysis (ESA) and Motor Current Signature Analysis (MCSA) work?
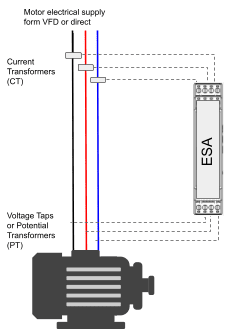
Electrical Signature Analysis (ESA)
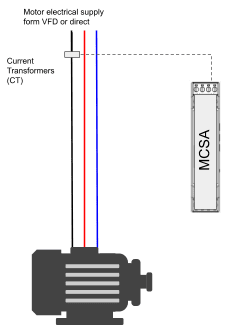
 Motor Current Signature Analysis (MCSA)
ESA requires monitoring of motor supply (stator) voltage supply and current on all three phases. This is illustrated in Figure 1. MCSA requires monitoring of motor supply current (stator) on one of the phases. This is illustrated in Figure 2. Motor stator windings are used as sensors in ESA and MCSA.
Any mechanical issue in the motor, transmission and the load lead to the air gap being disturbed. This leads to minor changes in the induced current signals from the rotor and also ripples on the voltage signal. Motor current is sensed by a Current Transformer (CT) while supply voltage is sensed via direct voltage measurement or through a Potential Transformer (PT). PT are only used in case of Medium and High voltage applications.
A precision and high speed data acquisition system is used to record time domain signals of current and voltage. These signals are then converted in the frequency domain. These time domain signals are sinusoidal with many harmonic components. These harmonics are present due to various electrical and mechanical fault conditions present in the motor, transmission and its load. These harmonics are produced due to change in air gaps, magnetic field and inductance of the motor due to these defects. These appear in the motor supply current spectrum as sidebands around supply frequency or the rotor slot passing frequency.
Key requirements for data collection:
- Data is captured between 0-6 kHz (minimum 15 KSPS sampling rate)
- Motor running steady at a load > 70%. Motor starting current for inrush current analysis
- CT/PT for data collection should be installed post VFD inverter
How does Motor Current Signature Analysis (MCSA) compare to Electrical Signature Analysis (ESA)?
MCSA requires current sensing on one phase while ESA requires collection of current and voltage data on all three phases. While MCSA can only provide information on faults. ESA can provide information on supply issues, imbalances etc. ESA data can be used for further analysis like Extended Park’s Vector Approach (EPVA) and Instantaneous Power Signature Analysis (IPSA), Pump Best Efficiency Point (BEP). Infact MCSA is a subset of ESA. ESA technique also allows detailed analysis of energy consumption and pump efficiency, which is not possible with MCSA alone.
What faults can be detected by ESA and MCSA?
The following faults can be detected:
- Electrical supply issues
- Broken rotor bars
- Air gap eccentricity
- Shorted turns in stator windings
- Stator electrical imbalance
- Mechanical imbalance or misalignment
- Bent shaft
- Bearing failure
- VFD issues
- Insulation degradation
- High connection resistance
- Impeller, blade or vane failure
- Clogging, cavitation, gas slug and gas lock
- Coupling, gearbox, belt and chain failure
- Seal and Coupling issues
Which assets are best suited for Predictive maintenance using ESA?
Any asset driven by an electrical motor can be used:
- Induction motors
- Electrical Submersible Pumps (ESP)
- Pumps
- Fans
- VFD
- Gear Boxes
What are the fault detection techniques used in ESA?
The following fault detection techniques are used as part of ESA:
- Motor Current Signature Analysis (MCSA)
- Current Spectrum Analysis (CSA)
- Demodulated Current Spectrum Analysis (DCSA)
- Extended Park’s Vector Approach (EPVA)
- Instantaneous Power Signature Analysis (IPSA)
- Wavelet Analysis
- Transient or Inrush Current Signature Analysis (Motor starting current)
What are the benefits of combining vibration monitoring with ESA?
Combining ESA data with data from vibration and ultrasound sensors, like C100X, can increase the accuracy of predictions. Vibration is more accurate in detecting load and transmission side faults, while ESA is more accurate in detecting motor and electrical supply defects. They together provide a holistic assessment of your asset reliability and efficiency.
How is IoT and AI being used in ESA?
Data collection for ESA or MCSA is very time consuming. IoT can enable automatic data collection. IoT also reduced risk of electrical shocks during data collection. It also allows data collection on a daily basis instead of a monthly or occasional manual data collection. It eliminates data collection errors due to improper procedure or human error. IoT enabled regular and accurate ESA data collection can improve prediction accuracy and timeliness.
Electrical Signature Analysis (ESA) requires deep expertise and experience in analysis. Even for an expert it requires considerable time to analyze this data. Now imagine this being done for all your motors on a daily level. This can be impossible due to manpower constraints. AI based systems like ioEYE Predict can help reduce this effort. AI systems analyze the data and when they detect an anomaly or a fault developing they can alert the expert. Who can then perform a detailed analysis using the various fault analysis techniques mentioned earlier in this blog. These techniques are now possible on the browser with products like ioEYE Predict.
What are the benefits of an IoT and AI powered ESA reliability solution?
- Reduced data collection efforts and costs
- Accurate and consistent data collection
- Regular and more frequently data collection for faster fault detection
- Automated data analysis with AI means reduced man hours spent in analyzing non fault data. With this time saved your team can expand the predictive maintenance program across more assets.
- Reduced safety issues with data collection from electrical supply lines